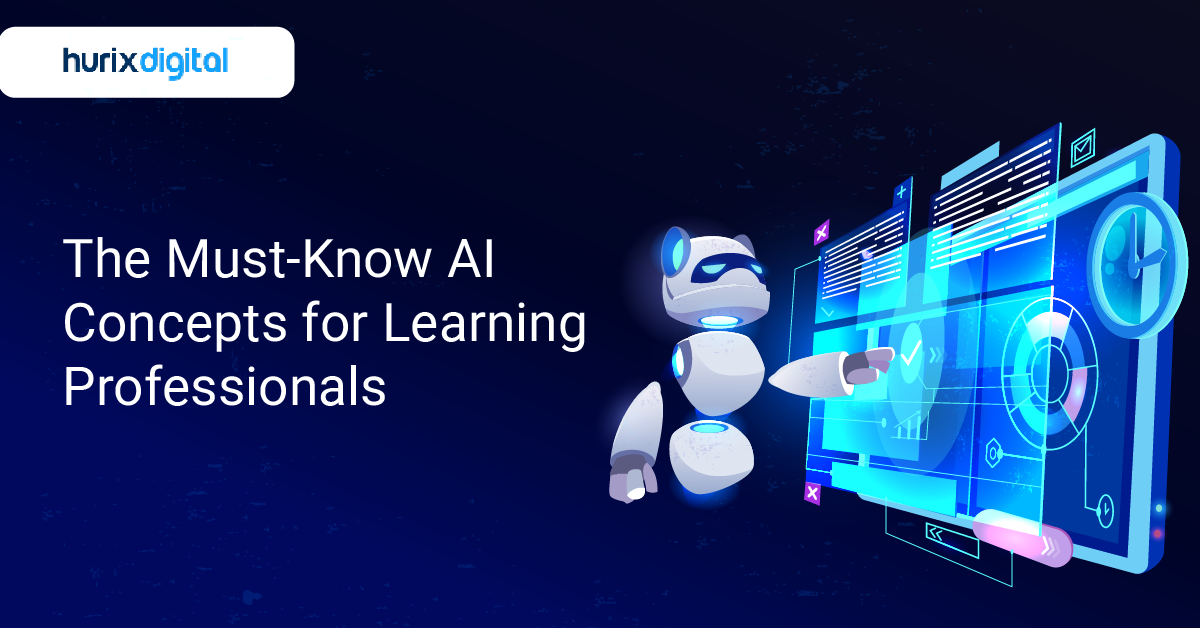
The Must-Know AI Concepts for Learning Professionals
Summary
AI redefines learning and development by automating training, personalizing learning paths, and addressing workforce challenges. This guide explores AI’s role in L&D, key AI concepts for learning professionals, and the latest trends in workforce development, helping organizations stay ahead.
Developments in AI technology have raised several concerns about their impact on businesses and their workforce. Since the 1980s, technological development has displaced workers faster than it has created jobs. However, it is worth noting that 60% of workers are employed in jobs that did not exist in the 1940s. While technological change can be daunting, leaving workers in specific roles and fields redundant opens up new opportunities for innovation.
This is where workforce development plays a crucial role. Workforce development means offering your employees opportunities for training, development, and continuous education, assisting them in upskilling or reskilling, and progressing in their careers.
The impact of AI and automation has set in motion trends in workforce development that aim to bridge the gap between automation and the human touch and address employee training needs in this new age. Further, AI in workforce development also addresses the increasing employee turnover with higher quitting rates, a wave of job-shifting, and the growth of hybrid work that emerged after the COVID-19 pandemic.
In this blog, we will explore the impacts of AI on L&D challenges and solutions, highlighting innovative ways that utilize machine learning to enhance the effectiveness of training programs.
Table of Contents:
- The Rise of AI in Learning and Development
- How to Overcome L&D Challenges with AI?
- AI-Powered Learning Solutions
- Machine Learning in Corporate Training
- Key AI Concepts for Learning Professionals to Improve Skill and Knowledge
- What to Foresee in the Future of AI in Training and Development?
- Conclusion
The Rise of AI in Learning and Development
AI has become a driving force in shaping the field of corporate training. This rapidly advancing technology is not just a trend but a major shift in how corporations approach L&D challenges.
Let’s dive deeper into the key aspects of the use of AI for bettering L&D:
1. Automated Content
AI’s role in content creation involves analyzing individual preferences, historical data, and industry trends to generate optimized and relevant materials.
Algorithms ensure that the content is up-to-date and aligned with the business environment’s dynamic nature.
2. Personalized Learning Paths
Personalization becomes central to how AI crafts individual learning paths based on user progress. This ability to adapt ensures that learners receive content at their own pace, helping foster engagement and understanding.
AI-powered learning solutions are continuously evolving, adjusting to the learner’s experiences and preferences to create a truly effective learning experience.
3. Assessments and Feedback
Traditional assessments often fail to provide compelling, real-time insights. AI-powered automated assessments save time and provide immediate feedback, helping learners track progress and focus on improvement.
Continuous feedback loops create an iterative learning process, helping enhance the overall effectiveness of training programs.
How to Overcome L&D Challenges with AI?
AI helps corporations address longstanding challenges in Learning and Development. Let’s look at some specific areas where AI is making a significant impact:
1. Customized Learning for Individual Learners
Automated AI algorithms can analyze particular individual learning styles and preferences. Customized content creation ensures steady engagement and long-term knowledge retention.
Assessments that dynamically adjust difficulty levels based on the learner’s proficiency ensure a personalized learning journey.
2. Predictive Training Analytics
AI’s predictive analytics are pivotal in foreseeing learner performance and identifying potential challenges. Strategically using predictive analytics enables intervention strategies to prevent potential roadblocks in learning and upskilling.
Data-driven feedback loops become an essential part of the learning process to enable continuous improvement.
3. Adaptive Learning Paths
AI-driven adaptive learning algorithms offer dynamic learning paths to cater to individual skill levels. Real-time adjustments made to training modules ensure that individual learning courses always meet specific evolving needs.
Increasing adaptability enables training programs to respond dynamically to ever-changing business requirements.
AI-Powered Learning Solutions
The influence of AI extends beyond theoretical concepts, creating practical and engaging learning offerings for corporate training.
1. Virtual Reality Simulations
Virtual Reality (VR) helps corporations create immersive experiences that simulate real-life scenarios for practical training in a safe and productive environment. Experiential learning encourages better retention through practical experiences so that knowledge is not simply taken in but deeply ingrained.
This has caused organizations to reconsider their approach to hands-on learning as VR-based training becomes easier to use due to its increasing cost-effectiveness and reliability.
2. Instant Learning Support
AI-driven chatbots and teachings offer immediate support for queries, helping remove barriers to instant learning. The availability of 24/7 learning increases accessibility to ensure that individuals can seek specialized assistance whenever needed.
AI’s ability to process natural language commands makes interactions more seamless and user-friendly.
3. Gamification of Training Modules
Gamified elements in learning modules foster fun in training and help increase engagement. Achievement badges and rewards can serve as motivational tools, encouraging continuous participation and a prolific attitude.
A healthy sense of competition can help facilitate a dynamic learning environment, enhancing the overall training experience for all learners.
Machine Learning in Corporate Training
Machine Learning in corporate training introduces previously unprecedented advancements:
1. Dynamic Course Recommendations
ML algorithms can analyze learner data to recommend more relevant and specialized courses. Refinement based on user feedback helps ensure that course recommendations don’t stagnate over time.
2. Automated Skill Analysis
ML can identify skill gaps through detailed data analysis. Customized training plans help address specific upskilling needs, aligning learning objectives with particular goals.
3. Predictive Maintenance
ML algorithms can conduct proactive analysis and resolve many potential technical issues. ML ensures a smooth learning experience with minimal disruptions to maintain the functionality of corporate learning platforms.
Key AI Concepts for Learning Professionals to Improve Skill and Knowledge
Here are 11 key AI concepts for learning professionals to improve their skills and knowledge:
1. Machine Learning
Machine learning lies at the heart of AI, empowering systems to learn from data and improve over time without explicit programming. Specialists in this field must understand the different types: supervised learning, unsupervised learning, reinforcement learning, decision trees, neural networks, and support vector machines, among other common algorithms.
Once you know what they are capable of doing, it becomes possible to create adaptive systems for teaching or recommendation engines for personalization that will optimize results based on predictions made by predictive analytics models using ML.
2. Natural Language Processing (NLP)
Natural Language Processing enables computers to understand human speech or writing so they can communicate with people more effectively. There are many ways educators might apply NLP.
This includes generating chatbots that respond in natural language to learner queries or analyzing large bodies of text for insight extraction towards intelligent tutoring system development. These programs would be able to understand questions learners ask and express naturally.
3. Deep Learning
Deep learning, a branch of machine learning (ML), centers around artificial neural networks with multiple layers that allow for complex data representation. Understanding deep learning allows educators to create advanced AI applications.
Some of them are speech recognition systems, image classification algorithms, and models for natural language understanding, thus changing the way we deliver and assess content.
4. Computer Vision
Computer vision enables machines to interpret and understand visual information from their environment. With vision and cognitive computing techniques, teachers can create immersive virtual reality (VR) and augmented reality (AR) learning experiences.
Moreover, they can automatically tag and classify content and improve assessment through technologies like facial recognition or emotion detection.
5. Recommender Systems
Recommender systems use AI algorithms to analyze user preferences and behavior and give them personalized recommendations on what to consume next. Educators need to learn how recommender systems work.
That is how they can design adaptive learning platforms, curated learning pathways, and targeted content delivery strategies for individual learners based on their needs and interests.
6. Generative Adversarial Networks (GANs)
GANs comprise two neural networks: the generator and the discriminator. These networks learn by competing against each other, which helps them produce realistic synthetic data.
For example, GANs can be used in educational programs to create virtual laboratories. They can also generate training sets for unusual cases or those that require privacy. Moreover, content developers may find them useful when creating lifelike videos, texts, or images.
7. Ethical AI
AI ethics is a top concern when working with educational systems. Teachers must account for fairness issues tied to algorithmic bias and student privacy vis-à-vis machines’ use of their personal information.
Additionally, moral questions surround automated decision-making through AI in learning and development environments. To ensure equal opportunity and gain students’ confidence in these programs, instructors need to follow ethical guidelines while being transparent about their intentions.
8. Data Privacy and Security
As AI-powered learning technologies become more common, protecting the privacy of student data and ensuring cybersecurity have become essential duties for educators.
Awareness of data protection regulations, such as GDPR, and implementing strong security measures can help reduce the risk of breaches or unauthorized access while keeping learners’ trust intact.
9. Explainable AI (XAI)
Explainable AI aims to clarify artificial intelligence models so that different stakeholders can easily understand how decisions are made based on them.
Learning professionals should prioritize XAI techniques since they enable students to learn more about themselves through assessments made by algorithms driven by this system. This system also recommends personalized AI in learning and development materials, thus enhancing comprehension and trust in an environment where machines do everything.
10. Transfer Learning
Transfer learning refers to when the knowledge gained from one task is applied to another related task, thereby speeding up AI learning and development and improving how models generally work.
Resource allocation optimization and adaptation of pre-trained AI models may be necessary to match specific educational settings, among other things. Transfer learning methods can achieve this, allowing teachers to maximize knowledge sharing across different domains.
11. Continuous Learning and Adaptation
Due to the fast-changing nature of AI and education, it is important to adopt a culture of continuous AI in learning and development. This will help experts in the field keep up with current trends, new technologies, and best practices.
By participating in career advancement activities, working with teams from various disciplines, and trying out novel methods, professionals can improve their skills with artificial intelligence’s progress, thereby fostering innovation within teaching institutions.
What to Foresee in the Future of AI in Training and Development?
The future of AI in learning and development is expected to feature an even more personalized and efficient training experience, broader accommodation in the academic Sector, and an even more significant impact on the learning and development industry.
The key statistics of AI for 2024 state:
- The global AI market is worth $150.2 billion and is expected to rise as 2024 reach its peak.
- The market for AI is projected to increase by 36.8% from 2023 to 2030 at a CAGR.
- In a global survey, a dominant 83% of businesses prioritize integrating AI into their core processes.
Conclusion
Integrating AI and automation into workforce development is not just a response to technological change but a proactive strategy to empower employees, foster innovation, and remain competitive in a rapidly evolving world. Embracing these trends can help organizations bridge the gap between automation and the human touch, creating a more dynamic and adaptable workforce prepared for the challenges and opportunities of the future.
Therefore, by being proficient in machine learning (ML), deep learning (DL), and Natural Language Processing (NLP), among other foundational ideas about artificial intelligence (AI), teachers will be able to design life-changing educational experiences that empower learners while catalyzing a company’s growth, thus shaping future education.
Hurix Digital offers workforce learning solutions and management systems for companies and educational institutions. Our corporate learning solutions and training technologies cater to diverse requirements. In addition to leading in-class technology and software infrastructure, we offer uniquely customized digital solutions.
Get in touch with us to know more!
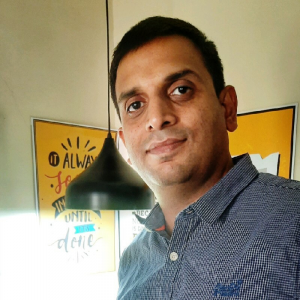