How Image Labeling Revolutionizes Machine Learning Models!
Powering most of the current cutting-edge technologies, most of us have heard and interacted with products of Machine Learning.
As a subset of artificial intelligence, which is expected to reach a market value of $204.30 billion by the end of 2024, machine learning is used to enable digital devices to learn and improve their processes. Through algorithm models and training data, many futuristic software, applications, robots, and intricate tools are born.
This piece will help you understand how to drive quality machine learning through one of its most crucial training data elements: Image Labeling. Dive in to learn about image labeling, its types, and how it affects the training data and, ultimately, the machine learning (ML) models itself.
Table of Contents:
- Why is Training Data So Important?
- The Fundamentals of Image Labeling
- Image Labeling Techniques
- How Image Labeling Drives Data Quality and Machine Learning
- These Pictures are More Than a Thousand Words
Why is Training Data So Important?
Before we get into image labeling, let’s quickly recap why training data is crucial.
Machine learning is made of algorithms, models, and data. To oversimplify, algorithms and models make up for the rules and logic that help define how learning happens; data make up for examples that these rules and logic train themselves. This training data helps the machine take reference and adapt to understand and process new unseen data.
Quality data helps systems generalize patterns, accurately predict outcomes, and make decisions. The higher the quality of the elements involved in the model, the lower its gap compared to the real-world outcome.
Also Read:
The Fundamentals of Image Labeling
Before we touch on how image labeling changes everything, let’s answer what is meant by image labeling.
Image labeling is one of the core parts of training data. It involves providing the algorithm and model datasets of images pre-defined with different classes, descriptions, etc. With the help of these images, the models learn to identify and address other new data.
Image labeling, also known as image annotation, is a vital part of numerous solutions that use machine learning, of which cutting-edge solutions are image identification, face recognition, retinal scans, autonomous vehicles, and even disease diagnoses.
Many of these solutions are used heavily in day-to-day life across numerous industries and in multiple activities. Ultimately, quality image labeling in training data leads to quality functions in ML models.
Image Labeling Techniques
Before learning what image labeling aspects to pay attention to, let’s dig a little deeper to understand the types or techniques of image labeling. Divided into five primary types, here are all the quick deets!
1. Class Labels
This broad classification of image labeling into categories provides foundational information about the image.
Here are some applications for this basic yet reliable technique:
- Class labeling allows models to sift through and organize information based on broad classification.
- Class label image training also allows the ML models to reduce initial processing time on irrelevant data.
2. Bounding Box Annotation
This image annotation technique involves drawing precise rectangles around objects within an image. When an image features multiple types of objects, this technique helps the ML model identify spatial location and facilitates object detection.
Reasons why it is crucial can be understood with these applications:
- Surveillance systems identify visitors or intruders with this technique.
- Autonomous vehicles detect obstacles and pedestrians.
- Retail software uses this data to manage product inventory better.
3. Semantic Segmentation
This technique delves deep into pixel-level image labeling. With data that contains details in pixels, ML models are trained to discern details, including object boundaries.
This image annotation technique is crucial when every bit of detail matters in the final solution. Here are some applications:
- It is used in medical imaging to identify and segment anatomical structures.
- Semantic segmentation is image annotation for deep learning and can be applied to autonomous navigation to understand environment and route details.
- When applied in satellite imagery, ML models can expedite urban planning.
4. Keypoint Annotation
A key point is an important dot in an image; with this image labeling technique, several key points are included in an image for the model to train and understand its relative position and even the meaning behind those positions.
Its concept is simple, and its applications are vast; here are some of its applications:
- Keypoint annotations of human joints in images help models identify their pose and stance. This can be used to discern generalized moods or even expressive intent.
- It can be used in facial recognition with pixel-level critical points of the eyes, ears, nose, and mouth.
- One cutting-edge application of keypoint annotation is that it can be used for ML models to help project holograms and other augmented reality purposes.
5. Captioning
Last but not least, the captioning technique assigns relevant text to describe the images. With this image label type in training data, the ML models can elaborate and help assign meaning to new images.
Here are some extremely significant and relevant applications of this image annotation:
- Accessibility is boosted as ML models describe images fed to them. They help overcome visual or cognitive impairments with descriptions.
- When sensitive or focused information is needed, ML models rely on captioning images as a part of their training data for algorithms to execute necessary content moderation.
How Image Labeling Drives Data Quality and Machine Learning
With these extremely relevant image annotation techniques and vast scope of application, image labeling influences a considerable part of the quality of training data that ML models depend on. Here are the five main ways the quality of image annotation for machine learning creates waves in the end product,
1. Improves Data Discernment
Image labeling has a direct impact on how well ML-modeled solutions discern data. With high-quality training data, especially in more focused applications like automated navigation and medical imaging, ML models can identify intricate data and discern patterns and analyses more accurately.
2. Optimizes Precision
Quality in image annotation techniques such as semantic segmentation and keypoint annotation is crucial, centered around precise boundaries and specific locations of critical points.
Focusing on databases with quality content helps ML models make more accurate conclusions. Without quality in image labels, the environment could be mapped wrong; if used in satellite imagery, it could lead to an unimplementable urban development plan.
3. Expedites Model Convergence
The point from which the ML model has sufficiently learned from the training data and can execute its goals accurately and reliably is model convergence.
The speed at which the model learns and adapts is significantly higher. With high-quality data, ML models can accelerate to the point of convergence much quicker, thus saving time and resources on the training phase of the solution.
4. Cultivates Better Generalization
When ML models are exposed to more diverse image labeling examples, they can more effectively adapt and generalize their learning.
With this, the end application is better equipped to cater to more unseen and general problems. The quality of image labeling drives the adaptability and performance of the model.
5. Eliminates Bias
Fairness and equity significantly impact the correctness of a solution. As image annotation is vetted and pristine concerning perspective, bias is eliminated by ensuring that annotations are fair, representative, and unbiased.
Though a meticulous and time-consuming approach, it prevents the perpetuation of biases in machine learning models.
Also Read: Cultural Influences on Typography: Global Perspectives in Typesetting
These Pictures are More Than a Thousand Words
As we have seen through this blog, training data forms the backbone of the ML model. There is no question now that machine learning has vast applications and is ultimately responsible for their performance.
It is through delivering high-quality image labeling that we can ensure the solution we seek to implement a bias-free, precise, adaptable ML application that can provide self-sufficiently.
All this, undoubtedly, is a challenge to go through on your own. That is why you can trust Hurix Digital to help develop digital solutions driven by quality data!
We know you’re raring to take advantage of this simple yet critical aspect of training data in your machine-learning models, so don’t hesitate! Contact us now!
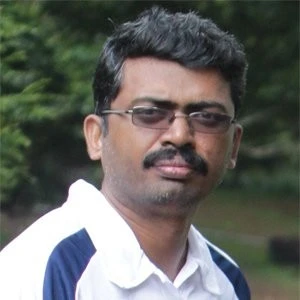
Gokulnath B is the Associate Vice President – Editorial Services. He is PMP, CSM, and CPACC certified and has 20+ years of experience in Project Management, Delivery Management, and managing the Offshore Development Centre (ODC).