Unlocking the Power of AI: Exploring Image Annotation for Enhanced Visual Understanding
Images are essential in this digital age of communication because they allow us to graphically represent feelings and information that are not limited by the limitations of human language.
Furthermore, machine and deep learning algorithms can interpret the visual material with high accuracy if the image attributes are properly categorized and labeled. AI image annotation has the potential to further advance this revolutionary technology that promotes improved visual comprehension.
Table of Contents:
Understanding Image Annotation
Image annotation is the addition of metadata to the image’s dataset. Annotation tools and text are used for the classification and labeling of data features in images. These features are then fed to deep learning and machine learning (ML) algorithms to train them.
During annotation, you tag, transcribe, and process the image to highlight the features that you want the ML models to recognize. After the deployment of the model, it can intuitively identify relevant features in non-annotated images to facilitate better decision-making for initiating subsequent actions.
In simple annotation, a phrase typically describes the object of the image. For instance, the image of a dog can be tagged as a ‘pet dog’.
In complex annotation, different entities or areas of the image can be tracked, counted, and identified. The unique objects of the image can be labeled. For example, the various breeds of dogs in an image can be isolated and tagged.
Also Read: Anatomy and Physiology Explained: Using Video-Based Modules for Medical Students
Discovering AI’s Potential for Precise Image Annotation
The meaningful description of images’ visual content for efficient indexing and retrieval is being taken to the next level with AI. AI is facilitating automated image recognition for large batches in an expeditious and error-free manner. AI models factor in the semantic reference and visual attributes of images for spontaneously generating annotations.
1. Techniques for AI-Based Image Annotation
AI models can leverage ML, NLP (Natural Language Processing), Computer Vision, and other techniques for image analysis and isolating relevant details. The details pertain to different image elements, such as humans, animals, text, structures, etc.
Optical character recognition, face recognition, scene segmentation, and object detection techniques are taught to AI models through training data for identifying image elements. The models can tag, use captions, and generate keywords to tell about the image theme in metadata or natural language format.
2. Different AI-Driven Image Annotation Types
Through supervised learning, AI or ML models can be trained to annotate image elements accurately. Interestingly, image annotation through AI takes various forms, as outlined below.
- Object Recognition
The AI model is trained to detect the availability, place, and occurrence of the pre-defined object inside an image to facilitate correct labeling. Once the process is repeated with various images, the model autonomously starts identifying objects with polygons, bounding boxes, and other such techniques.
Multi-frame data available through MRI or CT scans in healthcare can be annotated in a continuous stream. Trained AI models can accurately signify the presence of disease symptoms, such as cancer tumors, and their changing appearances over time.
- Image Classification
In this annotation type, AI models can detect if similar types of objects are present in unlabeled images across the complete set of data.
The model prepares the unlabeled digital image for tagging, analyzes it, and recognizes objects similar to those in other labeled images. It then classifies all relevant objects inside the image.
- Segmentation
The image’s visual content is analyzed to ascertain the similarities or differences between objects. Differences arising over time can be identified.
In semantic segmentation, the AI model groups similar objects and identifies them with the same label. The location, availability, and dimensions of the objects inside the image can be tracked. This is used for objects that are not required to be counted or detected individually across different images, as the dimensions may not be accurately revealed in the annotation.
For instance, if multiple images of a cricket field are being annotated, the spectators can be segmented from the cricket field with semantic segmentation.
With instance segmentation, the object class of the image is annotated. The dimensions, location, and availability of objects in visual content are tracked.
In the aforesaid example, each spectator on the cricket field can be labeled to find out the number of viewers enjoying the game. The AI model is capable of labeling each pixel within the image outline. Alternatively, coordinates on the border of the outlined area can be counted with boundary segmentation.
The instance and semantic segmentations are blended by panoptic segmentation for labeling both the objects and the background in an image. With AI models, changes to the characteristics of imaged areas can be detected over time.
- Boundary Recognition
AI models can be trained to identify object boundaries or its delineating lines in any image. A boundary is an edge that defines the object’s shape and can be in the form of human-drawn lines, splines, or topographic areas. With AI image annotation, the boundaries of objects in unlabeled images can be recognized easily.
In revenue maps, for example, the berms representing the segregation of individual lands can be identified. The AI models deployed in drones guide them on a safe course by steering clear of potential hindrances. With AI’s efficient boundary recognition capability, image annotation can be leveraged to identify cell-related abnormalities in medical imaging, separate the image’s foreground from the background, define exclusion zones in restricted areas, and do more such subtle tasks.
3. AI Image Annotation Techniques
The AI image annotation methods leverage different techniques based on the features made available by annotation tools. These techniques help in image classification and object recognition with a high degree of certitude, as discussed below.
- Landmarking Technique
The characteristics of the recognized object in an image are annotated with this technique. A trained AI model can, for example, identify the facial expressions, emotions, and features in the detected human face.
The pose-point feature allows annotation of the alignment and position of the body. In a game of cricket, for instance, the batsman’s hand grip over the bat can be determined when he hits a boundary.
- Bounding Box Technique
The AI model singles out the object by drawing a 2-D or 3-D box around it. The technique works optimally for symmetrical objects where occlusion doesn’t matter much.
- Polyline Technique
Plotting lines in continuity is used to annotate open shapes like power lines, which can have multiple segments.
- Masking Technique
The AI model can facilitate zooming in on the area of interest in an image by annotating it at the pixel level. The focal point of the image is revealed by masking other irrelevant areas.
- Transcription Technique
The AI model uses this technique for annotating text in multimodal datasets where visual elements are interspersed with texts.
- Tracking Technique
This annotation technique leverages the interpolation method for labeling and plotting the movement of objects across different video frames. The AI model starts with labeling a frame and then skips to a different frame for annotation.
With interpolation, the model tracks the movement of an object in intervening, unannotated frames to complete the motion path.
- Polygon Technique
The AI model annotates the edges of the irregularly shaped objects by marking their highest vertices.
Also Read: Ethical Considerations in the Evolution of AI Copy Editing: Charting the Future Path of AI Services
Moving Ahead
AI image annotation facilitates the processing of large datasets without any biases or errors. The AI models can be adapted to various domains, however complex, to accomplish annotations quickly and efficiently.
The recent advancements in AI models, which have been extensively trained through supervised learning, help in reducing the financial and administrative expenses related to image annotation. This makes tagged images more accessible for different applications.
Hurix Digital is instrumental in enhancing the visual understanding of innate elements of images at a professional level with state-of-the-art AI-driven image annotation tools. Through the efficient, quick, accurate, and economical retrieval and indexing of images in a flexible, scalable, and high-quality mode, our professionals are enabling artificial intelligence to reach its full potential.
Contact us for the deployment of AI models that are capable of annotating and tagging images swiftly and consistently without any human intervention.
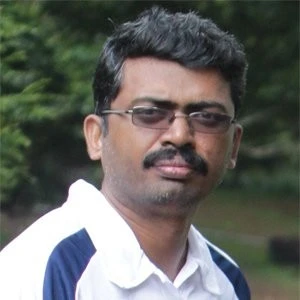
Gokulnath B is the Associate Vice President – Editorial Services. He is PMP, CSM, and CPACC certified and has 20+ years of experience in Project Management, Delivery Management, and managing the Offshore Development Centre (ODC).